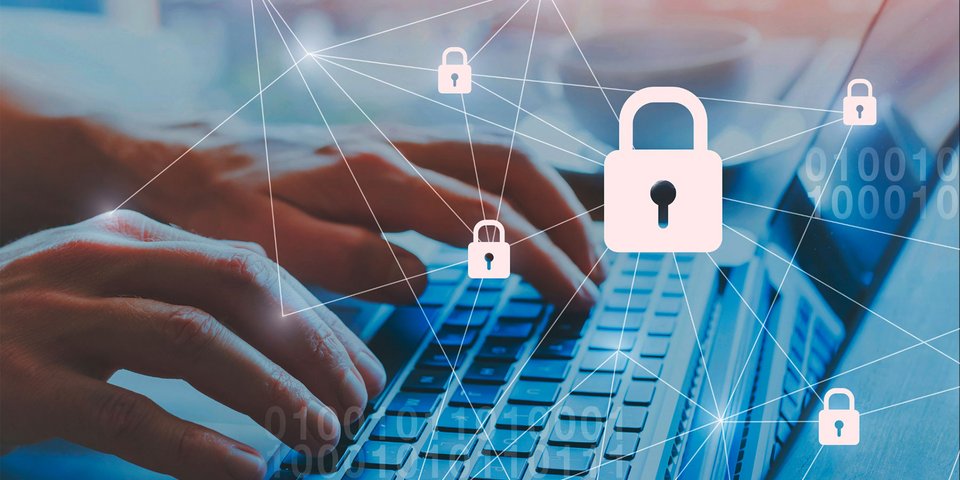
Data is not objective
ed* No. 02/2024 – Chapter 5
Probably the greatest challenge for ensuring safe AI systems lies in the provision of large, representative databases which are relevant to the respective issue and are used to train the underlying AI models. The quality and quantity of data are crucial for the performance and accuracy of AI models. There is a general consensus that neither AI applications nor the data used to train them should contain discriminatory elements or biases. But this is often difficult to avoid in reality. There is a possibility that unequal treatment that occurs in the real world is reflected in the data records without the operators of AI systems even knowing about it. Certain social groups or rare diseases, for example, are not adequately represented in existing data records. Such inaccurate or biased representations of reality harbour the risk that AI systems will not only reproduce but reinforce existing prejudiced decisions from the analogue world. This is particularly problematic when AI systems make decisions that have a direct impact on people’s lives, as is the case in the area of social insurance.
Against this backdrop, it is important to ensure that data is handled responsibly, as required by the General Data Protection Regulation1 also for the use of personal data for AI training. In addition, there must be transparency about how the AI systems work as well as their use, which is why transparency also plays a key role in the AI Act. However, it remains to be seen whether the provisions laid down therein are sufficient. This is because transparency regulations yield nothing as long as it is unclear how AI-based applications come to certain conclusions. Modern AI models with deep neural networks are often so complex that even developers sometimes find it difficult to explain the exact decision-making processes. There is also the question of the extent to which companies are prepared to disclose their AI models, as this could be seen as a loss of competitive advantage.
Data as the basis for transparency and trust
Nevertheless, only on the basis of transparency can people develop trust in AI systems, which increases the acceptance of AI applications, thus ultimately contributing to their successful and wider use. In order to maintain this trust, it is important to continuously scrutinise the further development of AI and correct it as and when required. In the best-case scenario, a balance between human judgement and AI systems can be achieved, ensuring ethical decision-making and accountability on the one hand, and greater efficiency, better resource management and more tailored provision of services on the other.
The value of personal data is constantly increasing as more and more high-quality data is required for ever more powerful and accurate AI models and applications. Since the start of the legislative process to establish a European Health Data Space (EHDS)– one of nine future cross-sector data spaces – health data has been at the centre of general interest at EU level. The EHDS is to enable better utilisation of health data for scientific research in the health and care sector – also explicitly for training, testing and evaluation of algorithms. In the case of sensitive health data in particular, it is crucial that insured persons have the opportunity to object to the disclosure of their personal electronic health data for secondary data use. For this reason, an objection regulation, the so-called opt-out, was introduced. The aim is to strike a balance between the needs of data users for comprehensive and representative data records – for example for AI-based research – and the preservation of people’s autonomy over their own health data.
Facilitation of data exchangeby the EHDS
The European Health Data Space (EHDS) is a cornerstone of the Euro- pean Health Union and the first common EU data space under the European Data Strategy. The EHDS aims to facilitate data exchange for the purpose of providing healthcare services across the EU (primary data use) and to create a coherent, trustworthy and efficient environment for research, innovation, policy-making and regulatory activities (secondary data use). In spring 2024, the European Parliament and the Council of the EU reached a political agreement on the European Commission's proposal onthe EHDS.